The significance of Large AI Models

This article has been co-authored by Dr. Rasmus Rothe, Dr. Johannes Otterbach and Eduard Hübner.
Large AI Models
Large AI Models, also known as Foundation Models, are dramatically changing Machine Learning (ML). These models differ from conventional Deep Learning (DL) models mainly through their size, the amount of training data and compute that are used to build them. They show various new behaviors and open up opportunities for research and industries.
At least since the release of OpenAI’s GPT-3, in May 2020, Large Models are widely accepted as a new paradigm in AI research. By now, there are many of them out there, like Megatron-Turing, Dall-E, T5, etc., and they are getting larger and larger. GPT-3 already has 175 billion parameters, which is more than 100 times more than its predecessor, GPT-2. In 2021, both Google, with their Switch Transformer, and the Beijing Academy of Artificial Intelligence (BAAI), with their Wu Dao, unveiled trillion-parameter models, thereby topping GPT-3’s size by an order of magnitude. We currently experience a race to the top with large consequences not only for research but also for industry. So far, Large Models mainly focus on NLP, but Solutions for other areas, such as computer vision, are on the horizon, as well as multi-modality. Stanford University established the Center for Research on Foundation Models (CRFM) as part of the Stanford Institute for Human Centered AI (HAI), and is demonstrating that Large Models are not just a research trend, but a new way of approaching AI.
Quality from Quantity
The technology behind Large Models is not much different from conventional ML/DL models. GPT-3, and comparable models, are based on Deep Neural Networks and Self-Supervised Learning. From an algorithmic perspective, Large Models include not much more than the DL technologies we knew before. Instead, the achievements of the models are grounded in a massive engineering effort that was necessary to deal with an unprecedented amount of data as well as addressing training stability challenges. Moreover, the amount of compute necessary to train Large Models lead to the convergence of industrial IT engineering and high performance computing. The resulting greater model size leads to a new quality of ML, usually summarized by the terms emergence and homogenization.
Emergence describes the phenomenon that certain properties arise inductively and spontaneously, without being explicitly designed or planned before. In the beginning of ML, emergence was limited to the way a task was performed by a model. Primitive ML models can infer from examples how they should solve a given problem. Building on that, Deep Learning enabled the extraction of high level features from data to identify the concepts relevant to humans. Large Models constitute the next step in this progression, such that new kinds of behavior and capabilities can emerge without being directly implemented by the developers. We can have intelligent behavior that emerges from (relatively) dumb algorithms, trained by lots and lots of data. GPT-3, for instance, is capable of adapting to a downstream task, of which it is given nothing but a natural language description and/or a few examples. This capability is called “in-context learning” and was neither pre-trained nor anticipated before it was discovered. Other examples of emergence include early arithmetic capabilities of models and broad multitasking. In summary, we can say that Large Models enable a new quality of AI, due to the emergence of novel properties.
Homogenization summarizes the trend that increasingly more tasks can be performed by employing fewer methodologies for building ML systems. Like in the case of emergence there is a progression since the start of Machine Learning. A decreasing number of models can be reused for an increasing number of applications. This is possible due to Large Models capabilities of zero- and few shot learning. Once the models are trained initially, they are able to learn new tasks without requiring more than a few examples. This adaptability makes it possible to solve tasks for which there is not much training data available. Therefore, although the training of Large Models requires tens of millions of dollars in compute, the models are expected to amortize the initial costs through their reusability. Overall we can see a functional reduction of many different models to fewer more general Large Models.
Within a very short time period, Large Models established themselves as a new paradigm in Artificial Intelligence. They come with their own Challenges in regards to technical infrastructure as well as to society and industries. The implementation and training of Large Models requires not only strong expertise in ML/DL but also a well set up environment for high performance computing, including the engineers supervising the hardware. This makes it, for now, only feasible for large institutions like MAAMA, to train their own Large Models. Considering the power of Large Models, their dependency on Resources and Ecosystem might lead to a massive power shift towards big tech, if we do not find a way to make Large Models and the related research open access. This is one of the reasons, why leading European AI companies have come together to work towards building their own Large Models, including the hardware infrastructure. These efforts will be channeled under the umbrella of the Large European AI Model initiative (LEAM). We will examine the reasons and the strategy behind this initiative in the next article.
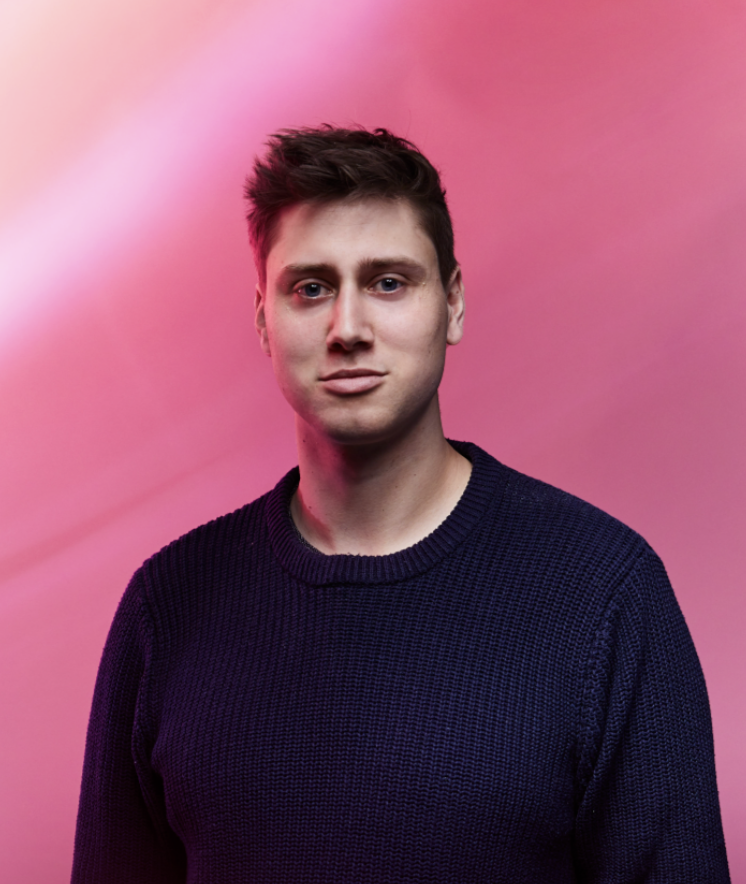
Read more

May 11, 2022