In an earlier piece, we already predicted that Open Source(OS) and the commoditization of AI would be a significant trend for this year. Since the landscape is rapidly evolving and proving pivotal for startups, we want to provide an overview of what happened recently and why it is crucial. Open Source is essential for companies who want to apply AI because it allows them to build their products independent of proprietary solutions. So far, however, OS models have been lagging behind in capabilities compared to models like GPT-4. A further difficulty for startups and SMEs has been the computing costs of fine-tuning and hosting an LLM. Both of these problems might become easier to solve than many people thought, as new research shows us how to get more capable OS models with less computing.
Microsoft surprises with a 13bn parameter model that shows capabilities similar to GPT-4
On June 5, Microsoft Research published a report on Orca, a 13bn parameter open-source model that is more than just another LLM with a fun animal name. The model shows benchmark performances comparable to GPT-4, despite being orders of magnitude smaller and not having been trained on the same data. The model instead has been trained to imitate GPT-4. As the authors state: "Orca learns from rich signals from GPT-4 including explanation traces; step-by-step thought processes; and other complex instructions, guided by teacher assistance from ChatGPT."
To understand how much of a breakthrough this was, one must recognize that merely two weeks earlier, a paper had been published arguing that imitating proprietary models (like GPT-4) was not possible. In the now outdated paper, the authors concluded: "... model imitation is a false promise: there exists a substantial capabilities gap between open and closed LMs that, with current methods, can only be bridged using an unwieldy amount of imitation data or by using more capable base LMs." The fact that this statement has already been proven wrong is an impressive demonstration of how fast knowledge is progressing in this field. This new development unlocks new possibilities for Entrepreneurs.
New Paper shows a way for near-lossless compression of LLMs
Compressing an LLM enables you to run it with much less compute and on much smaller devices. The fact that this paper demonstrates a way to do these compressions without a significant loss of quality should again make small companies hopeful. It might enable an extensive array of new products. The paper itself paints an impressive picture of the potential of this advancement: "This makes it possible to run 33B parameter LLM on a single 24 GB consumer GPU without any performance degradation at 15% speedup, thus making powerful LLMs available to consumers without any downsides." This development, again, is important news from a startup perspective because it makes LLMs much more accessible even with low amounts of compute.
Inverse scaling laws show that bigger is not always better
For a long time, it seemed that larger models would always be more capable due to the scaling laws of LLMs, and whoever could effort the most extensive amounts of computing would also have the highest-performing models. This tendency might have resulted in a massive power shift toward Big Tech, creating a "winner takes it all"-situation in AI. A new paper, however, shows that LLMs, in many ways, get worse with scale. The authors state:
"Our tasks have helped drive the discovery of U-shaped and inverted-U scaling trends, where an initial trend reverses, suggesting that scaling trends are less reliable at predicting the behaviour of larger-scale models than previously understood. Overall, our results suggest that there are tasks for which increased model scale alone may not lead to progress and that more careful thought needs to go into the data and objectives for training language models."
For startups and SMEs, this shows that there is much more room for small players to compete with products that come out of Big Tech.
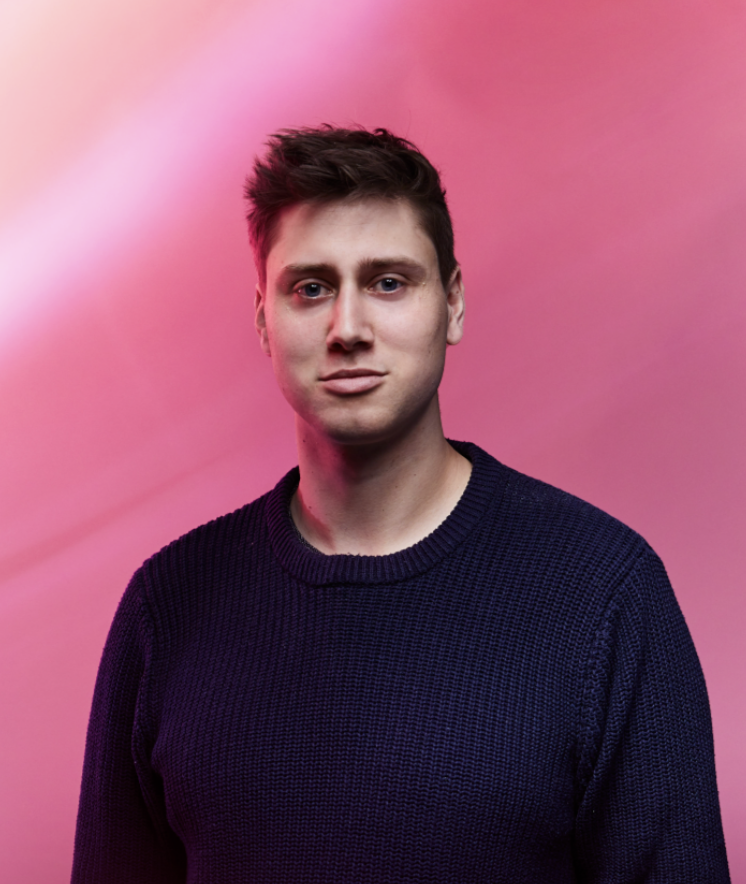